City-scale Sky View Factor
GENERAL INFORMATION
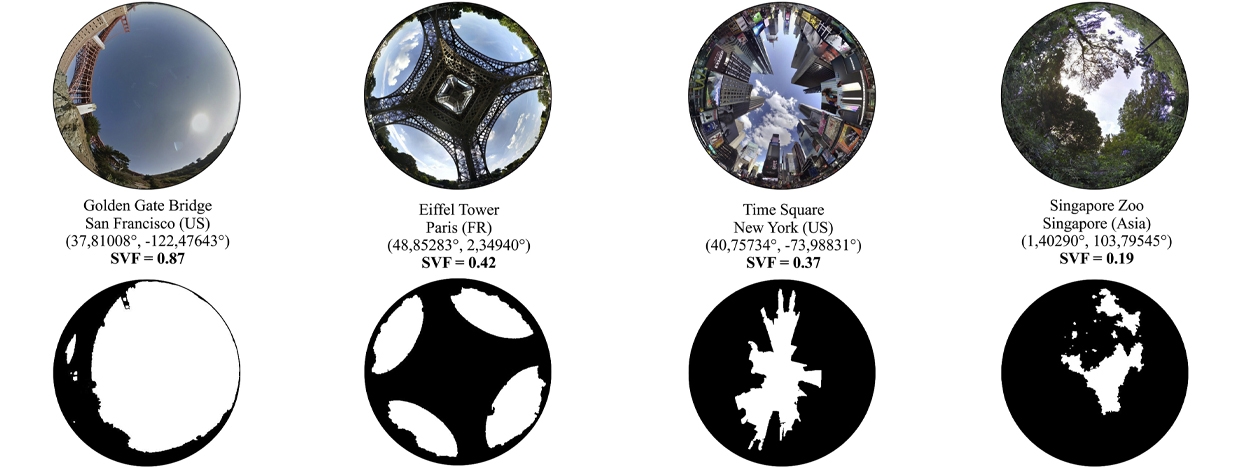
The Sky View Factor (SVF) is a geometric principle that plays a significant role in urban climatology. It is defined as the ratio of the radiation a planar surface receives to the radiation emitted by the entire hemispheric environment. In essence, it measures the proportion of the visible sky from a specific point.
This ratio is dimensionless, which means it is not associated with any physical units. It is a pure number that typically ranges from 0 to 1. A SVF of 0 signifies that the sky is not visible (as in a completely enclosed or indoor location), while a SVF of 1 indicates that the entire sky is visible (as in an open field or flat terrain).
The SVF is particularly valuable in urban environments, where the presence of buildings and structures creates intricate landscapes. For example, in urban street canyons (the spaces between buildings), the SVF measures the proportion of the visible sky or open space. A lower SVF in these canyons suggests a limited view of the sky due to tall buildings, whereas a higher SVF signifies a more open space.
By quantifying the degree of openness in an urban area, the SVF can offer valuable insights into various environmental factors such as sunlight exposure, wind speed, and temperature distribution. This makes it an essential tool for urban planning, design, and environmental studies, as it helps quantify the proportion of visible sky when looking upwards from a specific location within a built environment. It is a critical parameter in understanding urban microclimates, as it directly influences factors such as solar access, ventilation, and thermal comfort. Therefore, it is a key indicator used in designing energy-efficient buildings, improving urban ventilation, and enhancing the overall quality of life in urban spaces.
SVF AND THERMAL COMFORT
Different SVFs influence solar radiation impact and energy budgets in an urban environment. This is because SVF determines the proportion of the sky visible from a certain point, which, in turn, affects the amount of solar radiation that point receives. For instance, in locations with an SVF of 1, offering an unobstructed view of the sky, direct short-wave radiation from the sun and long-wave radiation from the atmosphere are received at the point, particularly under clear sky conditions. In contrast, if the location is situated in a valley or street canyon where the SVF is less than 1, the radiation and energy budgets are altered. This is due to multiple factors:
- Reflections: The surrounding surfaces reflect a portion of the incoming short-wave radiation, which can increase the total amount of radiation received at the location.
- Absorption and Re-emission: The surrounding surfaces absorb short- and long-wave radiation and re-emit it as long-wave radiation. This increases the amount of long-wave radiation received at the location.
- Decreased Outgoing Long-wave Radiation: Outgoing long-wave radiation from the ground is partially absorbed by the surrounding surfaces and re-emitted, reducing the amount of radiation that escapes to the sky.
The Sky View Factor (SVF) is also a crucial determinant of thermal comfort and air quality in urban environments, as it is linked to ventilation and temperature patterns. Urban environments with higher SVF values generally benefit from enhanced ventilation, promoting increased airflow. This increased airflow aids in the dispersion of heat and pollutants, improving air quality. However, the relationship between SVF and temperature can fluctuate across different times and locations.
Urban areas with higher SVF values typically undergo more effective cooling, especially during nighttime. In contrast, regions with lower SVF values, often characterized by taller buildings, denser urban layouts, or extensive tree coverage, cast deeper shadows throughout the day. These shadows provide a refuge from direct sunlight, leading to cooler temperatures within these shaded areas.
During the daytime, the correlation between SVF and temperature is also strongly influenced by the exposure and composition of urban canyons. Unfavorable exposure to solar radiation and a lack of trees can increase thermal discomfort. In this case, reduced sky view can limit longwave radiation exchange, increasing heat-trapping within urban canyons. Therefore, while SVF plays a significant role in shaping urban thermal comfort and air quality, its impact on heat varies depending on various factors.
MEASURE AND MODELLING OF SVF
Modeling the Sky View Factor typically involves using computational techniques to simulate the obstruction of the sky by surrounding buildings and landscape features. This can be achieved through various methods, including GIS-based analysis, hemispherical photography, deep learning, and computer vision. Advanced models may incorporate factors such as building heights, orientations, and surrounding topography to estimate SVF at different city locations accurately.
GIS-Based Applications. GIS-based numerical models are widely used for estimating SVF in urban environments due to their ability to handle large-scale spatial data and integrate various urban features. These models utilize 3D or 2.5D building databases and incorporate advanced algorithms to simulate the visibility of the sky from different locations.
Process:
- Data Integration: GIS-based models start with integrating spatial data, including 3D or 2.5D building models, terrain data, and land use information. These datasets provide a detailed representation of the urban environment.
- Digital Elevation Model (DEM) Creation: A DEM is created to represent the elevation of each point in the urban area. This model includes the height of buildings and other structures, which is crucial for determining sky obstruction.
- SVF Calculation Algorithms: Advanced algorithms, such as ray tracing or voxel-based methods, simulate the path of light rays from the ground upwards. These algorithms calculate the proportion of sky visible by tracing rays from multiple points within the urban area to the sky, accounting for obstructions by buildings and other structures.
- Spatial Analysis: The calculated SVF values are mapped across the urban area, producing a detailed spatial representation of sky visibility. This allows for identifying areas with varying degrees of sky openness, facilitating urban planning and design interventions.
Advantages:
- Comprehensive Analysis: Can handle large-scale spatial data, providing a detailed and comprehensive analysis of SVF across entire cities.
- Integration Capabilities: Easily integrates with other urban data, enabling multifaceted environmental analyses.
- High Accuracy: Advanced algorithms and detailed spatial data ensure high accuracy in SVF estimation.
Hemispherical Cameras. SVF can also be directly measured using ground-based cameras equipped with fisheye or panoramic lenses. These cameras capture hemispherical images of the sky from specific locations within the urban environment, providing precise and localized SVF measurements.
Process:
- Camera Deployment: Cameras are strategically placed at selected locations to capture a representative sample of different urban configurations.
- Image Capture: Fisheye or panoramic lenses capture wide-angle, hemispherical images of the sky, including obstructions such as buildings and trees.
- Image Processing: The captured images are processed to identify the sky and non-sky regions. This involves techniques such as thresholding to differentiate between sky and obstructions.
- SVF Calculation: The SVF is calculated by determining the ratio of visible sky area to the total hemispherical area in the image. This provides a quantitative measure of sky openness at a specific location.
Advantages:
- High Spatial Resolution: Offers detailed, localized SVF data.
- Real-time Monitoring: Enables continuous monitoring and assessment of temporal changes in SVF.
- Direct Measurement: Provides direct and precise measurements without relying on computational simulations.
Deep Learning and Computer Vision. Recent advancements in deep learning and computer vision have enabled the estimation of SVF from street-level imagery, such as Google Street View. These methods leverage large-scale image datasets and sophisticated neural networks to predict SVF accurately.
Process:
- Data Collection: Street-level images are collected from sources like Google Street View, covering various urban settings.
- Model Training: Convolutional Neural Networks (CNNs) are trained on labeled datasets with known SVF values. The model learns to recognize patterns and features that correlate with the visibility of the sky.
- Feature Extraction: The trained model identifies features within the images that affect sky visibility, such as the arrangement and density of buildings and the presence and height of trees obstructing the sky.
- SVF Prediction: The model predicts SVF values for new street-level images by analyzing the proportion of sky visible in the image. This involves calculating the ratio of sky pixels to the total number of pixels in the image.
Advantages:
- Scalability: Can process large volumes of imagery, making it suitable for city-scale analyses.
- Granularity: Provides detailed, location-specific SVF data.
- Accessibility: User-friendly applications can be developed, making SVF estimation accessible to non-experts.
Challenges:
- Data Privacy: Ensuring privacy when using street-level imagery.
- Model Generalization: Ensuring the model performs well across different urban environments.
TOOLS AND DATA
Various approaches and tools are used for modeling and measuring SVF in urban areas, including GIS-based applications, hemispherical cameras, and deep learning and computer vision techniques. Each approach utilizes specific software, tools, and data types to capture and analyze the visibility of the sky from different urban locations.
GIS-BASED APPLICATIONS
Software:
- SkyHelios: This software tool uses a digital elevation model (DEM) to calculate SVF by simulating the sky visibility at each point of the grid. It can handle complex urban topographies and provides high-resolution SVF maps.
- RayMan: This is a free available micro-scale model for calculating and estimating the Sky View Factor (SVF), sunshine duration, shadow, and, in general, radiation fluxes. In addition, RayMan is able to estimate the most used thermal indices used in human biometeorology.
- ArcView SVF Extension: An extension for ArcGIS that allows users to compute SVF using 3D urban models. It integrates seamlessly with other GIS functionalities, enabling comprehensive urban analyses.
- SOLWEIG (Solar and LongWave Environmental Irradiance Geometry): This model calculates SVF by considering solar radiation and shadowing effects. It uses a high-resolution 2.5D raster, also known as a Digital Surface Model (DSM), and can simulate the impact of different urban forms on thermal comfort.
Data:
- 3D Building Models: Detailed models of urban buildings, including their heights and shapes, which are crucial for accurately simulating sky obstruction.
- Digital Elevation Models (DEM): High-resolution elevation data that includes terrain and building and tress heights is necessary for creating accurate urban landscape representations.
- Land Use Data: Information on the types of land use (e.g., residential, commercial, green spaces) to provide context for SVF analysis.
- Aerial Imagery: High-resolution images taken from above to assist in validating and refining the 3D building and terrain models.
HEMISPHERICAL CAMERAS
Tools:
- Fisheye Lens Cameras: Cameras equipped with fisheye lenses that capture hemispherical images of the sky. These lenses provide a 180-degree field of view, ideal for SVF measurement.
- Panoramic Cameras: Cameras that capture wide-angle panoramic images, which can also be used to estimate SVF.
- Image Processing Software: Software such as MATLAB, ImageJ, or custom-built tools to process the captured images and differentiate between sky and obstructing elements. Techniques like thresholding and segmentation are used for analysis.
Data:
- Hemispherical Images: Images captured using fisheye or panoramic lenses from various locations within the urban area.
- Calibration Data: Information required to calibrate the cameras and ensure accurate measurements, such as camera height and angle of capture.
- Location Metadata: GPS coordinates or other location data to accurately map the SVF measurements to specific urban locations.
DEEP LEARNING AND COMPUTER VISION
Software:
- TensorFlow/PyTorch: Popular deep learning frameworks used to develop and train convolutional neural networks (CNNs) for image analysis and SVF prediction.
- OpenCV: A computer vision library that provides tools for image processing and feature extraction, useful for pre-processing street-level images.
- Custom Neural Network Models: Models specifically designed to estimate SVF from street-level imagery, leveraging architectures like CNNs.
Data:
- Street-Level Imagery: Images sourced from platforms like Google Street View or collected using mobile cameras. These images provide diverse urban views necessary for training and testing the models.
- Annotated Datasets: Datasets where images are labeled with known SVF values, used to train and validate the deep learning models. These annotations are crucial for supervised learning.
- Pre-trained Models: Deep learning models that have been pre-trained on large image datasets, which can be fine-tuned for SVF estimation tasks.
- Hardware Resources: High-performance GPUs for training deep learning models, which require substantial computational power.
MAIN APPLICATIONS
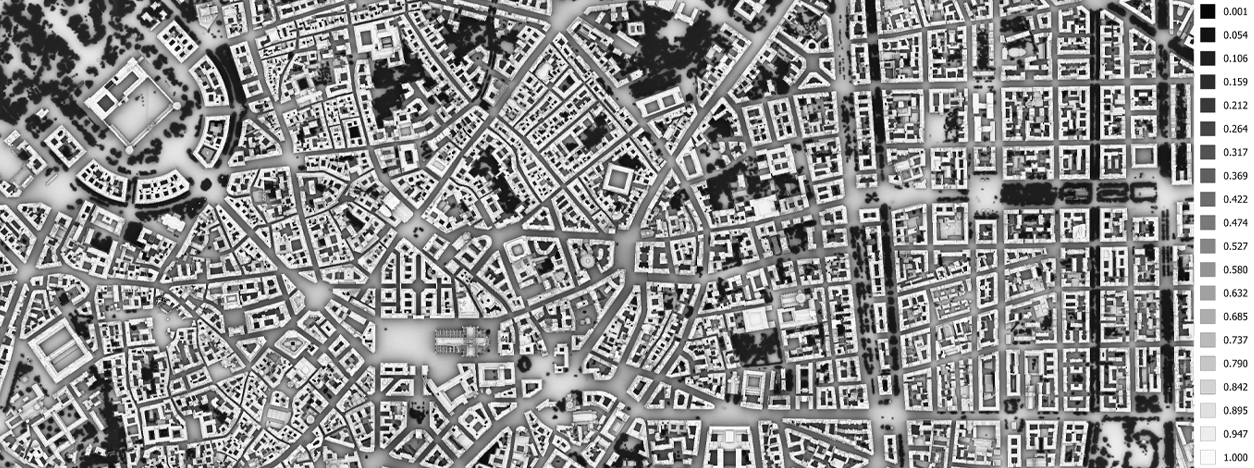
The Sky View Factor (SVF) is a critical parameter that has significantly advanced our understanding of urban climate relationships. Extensive research has explored SVF’s connection to various urban phenomena, including the urban heat island (UHI), thermal comfort, energy budgets, air temperature, and daylight availability. Below are detailed explanations of the main applications of SVF analysis:
- Outdoor Comfort Assessment: SVF analysis is essential for assessing outdoor thermal comfort, particularly in densely built environments. By evaluating how much of the sky is visible from street level, urban designers can create public spaces that maximize shade and wind flow, enhancing pedestrian comfort. This information is crucial for planning parks, plazas, and pedestrian pathways to ensure they remain comfortable even in extreme weather conditions.
- Climate Change Adaptation: SVF data is crucial for climate change adaptation strategies to mitigate the effects of rising temperatures and heat waves in urban areas. High-resolution SVF maps help identify vulnerable areas needing additional cooling interventions, such as increased vegetation, reflective materials, or innovative cooling technologies. This promotes resilient urban design and planning practices capable of withstanding future climatic changes. By analyzing SVF, urban planners can design green spaces, optimize building orientation, and strategically place shading elements to reduce heat retention in urban environments. Lower SVF values often correlate with reduced daytime urban heat island (UHI) effects, as more obstructions can lead to better shading and cooler microclimates. Higher SVF can facilitate urban ventilation at night, helping dissipate heat.
- Air Quality Improvement: Lower SVF values can indicate areas where air pollutants might be trapped due to poor ventilation. Understanding SVF distribution allows urban planners to design cities with better airflow patterns, helping to disperse pollutants and improve overall air quality.
- Solar Access Planning: By quantifying the amount of sky obstructed by buildings, SVF analysis informs solar access planning. This is vital for:
- Renewable Energy Generation: Ensuring solar panels receive adequate sunlight for optimal performance.
- Daylighting Optimization: Designing buildings to maximize natural light reduces the need for artificial lighting and thus lowers energy consumption.
- Energy Consumption Reduction: Minimizing heating and cooling needs by optimizing building placement and orientation to balance sunlight exposure.
- Transportation Planning: SVF analysis can inform the design of transportation networks by identifying shaded routes that are more comfortable for pedestrians and cyclists, encouraging active transportation, and reducing reliance on motor vehicles.
RELATED LINKS AND ADDITIONAL RESOURCES
- Comparison of models calculating the sky view factor used for urban climate investigations: This study compares different approaches to get SVF, including three methods (Steyn’s GIS-script, RayMan, BMSkyView) and three models (SkyHelios, ArcView, SOLWEIG).
- Method for calculating Sky View Factor using Google Street View: GSV is used to calculate the SVF to study its relation to physical elements. The SVF distribution showed higher values in quasi-residential areas and along major roads.
- Sky view factor measurement using a spherical camera: A spherical camera (RICOH Theta S) with a 360° field of view is introduced for SVF measurement, and its effectiveness is compared to fisheye cameras.
- A script for calculating the sky view factor for urban climate investigations: Models including SkyHelios, SOLWEIG, ArcView SVF-Extension, and manual methods like Steyn, RayMan, and BMSkyView are compared. A new GIS-based approach is introduced based on an ArcView script.
- RayMan and SkyHelios Model: RayMan and SkyHelios are two free micro-scale models available for calculating and estimating the Sky View Factor (SVF), sunshine duration, and shadow and radiation fluxes. In addition, RayMan can estimate the most modern thermal indices used in human biometeorology.
- SkyHelios Software: A software tool for calculating the Sky View Factor (SVF) and other urban climate metrics, useful for researchers and urban planners.
- Sky view factor analysis – implications for urban air temperature: This study links the sky view factor to urban air temperature variations, using fish-eye photographs taken at different heights. It finds a strong relationship between SVF and air temperature.
- Review of methods used to estimate the sky view factor: This review outlines methods for calculating SVFs, including geometric, fish-eye photography, GPS, 3D modeling simulations, and big data approaches using street view images.
- Solar radiation and daylighting assessment using the SVF: This study introduces methods to assess solar radiation, daylighting availability, and SVF modifications in complex urban environments. It combines numerical methods, 3D simulation software, and photo processing using digital cameras with special mirrors.
- A 3D-GIS extension for sky view factors assessment: This paper presents a Geographic Information System (GIS) 3D extension that is a tool to simulate the representation of sky view factors (SVF) while calculating their values.
- SVF-tools on GitHub: A GitHub repository for SVF-tools, providing open-source resources for calculating the Sky View Factor (SVF).
- Sky View Factor footprints for urban climate modeling: This study introduces a Big-Data approach to calculate SVFs for urban areas from Google Street View (GSV).
- Sky View Factor Calculation. A computational-geometrical approach: This study aims to save the time associated with manual methods of calculating SVF by developing a Rhino-Grasshopper component to quantify them via the hemispheric projection of a 3D model.
- Sky view factor calculation and its application in urban heat island studies: This study aims to determine the sensitivity of the SVF and its impact on the UHI.
*Images from
https://www.sciencedirect.com/science/article/pii/S2212095518301883