Land Cover Classification
GENERAL INFORMATION
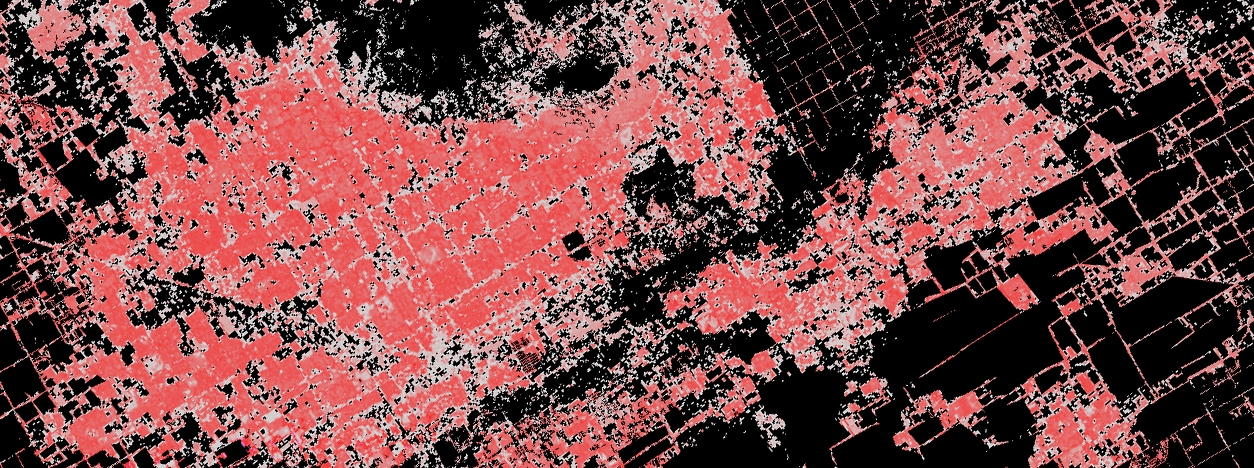
Land cover refers to the physical characteristics of the Earth’s surface, encompassing vegetation, water bodies, and human-made structures. It includes various features like forests, grasslands, wetlands, lakes, rivers, and cities. Accurate land cover information is crucial for diverse applications, including natural resource management, urban planning, and environmental monitoring. Understanding the distribution and characteristics of different land cover types empowers decision-makers to make informed choices regarding the sustainable management and protection of the Earth’s natural resources.
Remote sensing is a powerful and efficient tool for land cover classification, providing essential information for land resource management, cultivated area evaluation, and economic assessment. It enables the accurate identification and categorization of various land cover types over large areas. By utilizing advanced remote sensing techniques, such as active and passive sensors, different land cover types can be efficiently identified and analyzed.
Active sensors, including radar and lidar, emit their own energy and measure the reflected energy that returns to the sensor. On the other hand, passive sensors measure the naturally emitted or reflected energy from the Earth’s surface or atmosphere, using optical sensors to detect visible, near infrared, and shortwave infrared light. These remote sensing techniques play a vital role in mapping and monitoring land cover changes over time, providing valuable insights into the impacts of human activities on the Earth’s natural resources.
By leveraging remote sensing techniques and analyzing land cover data, we gain a comprehensive understanding of the Earth’s surface, enabling us to make informed decisions and promote responsible land use practices.
MAIN REMOTE SENSING TECHNIQUES USED FOR LAND COVER CLASSIFICATION
Land cover classification relies on a range of remote sensing techniques, each offering unique capabilities and insights. These techniques include:
- Multispectral Imagery: Multispectral sensors capture imagery in multiple specific wavelength bands, carefully selected to target key features of interest. By analyzing the unique spectral responses of different materials, such as vegetation, water bodies, and built-up areas, accurate land cover classification can be achieved. Multispectral imagery provides valuable insights into the distribution and characteristics of different land cover types.
- Hyperspectral Imaging: Hyperspectral sensors collect imagery in numerous narrow and contiguous spectral bands, providing detailed information about the chemical and physical properties of the Earth’s surface. This high spectral resolution enables the detection of subtle differences in land cover types. By analyzing a broader range of spectral signatures, hyperspectral imaging enhances the accuracy and precision of land cover classification, particularly in complex environments.
- Synthetic Aperture Radar (SAR): SAR sensors operate by transmitting microwave signals and measuring the backscattered signals from the Earth’s surface. This technique is effective regardless of weather conditions and can capture data during both day and night. SAR can penetrate vegetation and clouds, allowing for the mapping of land cover types and providing valuable information about surface roughness, vegetation structure, and moisture content.
Digital image classification plays a crucial role in the land cover classification process. It involves assigning pixels or groups of pixels from a remote sensing image to specific land cover classes. The goal is to accurately classify each pixel into a single class (crisp or hard classification) or associate the pixel with multiple classes (fuzzy or soft classification).
While crisp or hard classification assigns each pixel to a single land cover class with no ambiguity, providing a clear-cut representation of land cover types, fuzzy or soft classification assigns membership values to pixels, indicating the degree of membership to different land cover classes, allowing for the representation of uncertainty and mixed land cover areas. Both crisp or hard classification and fuzzy or soft classification have their applications depending on the specific objectives and characteristics of the land cover classification project.
Some examples of applications for crisp or hard classification and fuzzy or soft classification:
Crisp or Hard Classification:
- Land use planning: Crisp or hard classification can be used to produce clear and unambiguous land cover maps for land use planning purposes. These maps provide a definitive representation of land cover types, allowing planners to make informed decisions about land use and zoning.
- Natural resource management: Crisp or hard classification can also be used to produce land cover maps for natural resource management. These maps provide a clear representation of different land cover types, allowing resource managers to assess the distribution and characteristics of natural resources and make informed decisions about their management.
Fuzzy or Soft Classification:
- Ecosystem assessment: Fuzzy or soft classification can be used to produce land cover maps for ecosystem assessment. These maps capture the uncertainty and gradual transitions between different land cover types, allowing for a more nuanced representation of ecosystem dynamics.
- Climate change modeling: Fuzzy or soft classification can also be used to produce land cover maps for climate change modeling. These maps capture the uncertainty and mixed land cover areas, allowing for a more accurate representation of the Earth’s surface in climate models.
In the context of climate change modeling, these fuzzy or soft classification maps can provide a more accurate representation of the Earth’s surface than crisp or hard classification maps. This is because they capture the gradual transitions and mixed land cover areas that are often present in the real world, allowing for a more realistic representation of the Earth’s surface in climate models.
For example, a fuzzy or soft classification map might show a pixel as being 60% forest and 40% grassland, rather than assigning it to a single land cover class.
This classification process leverages the spectral information captured by remote sensing techniques to delineate and categorize land cover features. By employing these remote sensing techniques and utilizing advanced image classification algorithms, land cover classification enables the comprehensive assessment and monitoring of land resources. This information is vital for environmental management, natural resource planning, and decision-making processes, allowing for informed choices regarding land use, conservation, and sustainable development.
MAIN SATELLITES USED FOR LAND COVER CLASSIFICATION
Many satellites are used for land cover classification, including PlanetScope, Landsat, Sentinel, MODIS, and others. These satellites can carry either active or passive sensors:
- Active Sensors: Active sensors, such as those found on the Sentinel-1 satellite, or LiDAR, emit their own signals and measure the backscattered energy. This technology is especially useful for applications requiring all-weather and day-and-night observations.
- Passive Sensors: Passive sensors, like those on the Landsat series (e.g., Landsat 8), measure the naturally occurring radiation reflected or emitted by the Earth’s surface. They provide information across various spectral bands and are widely used for land cover classification.
MAIN TECHNICAL FEATURES OF THE OUTPUT LAND COVER CLASSIFICATION
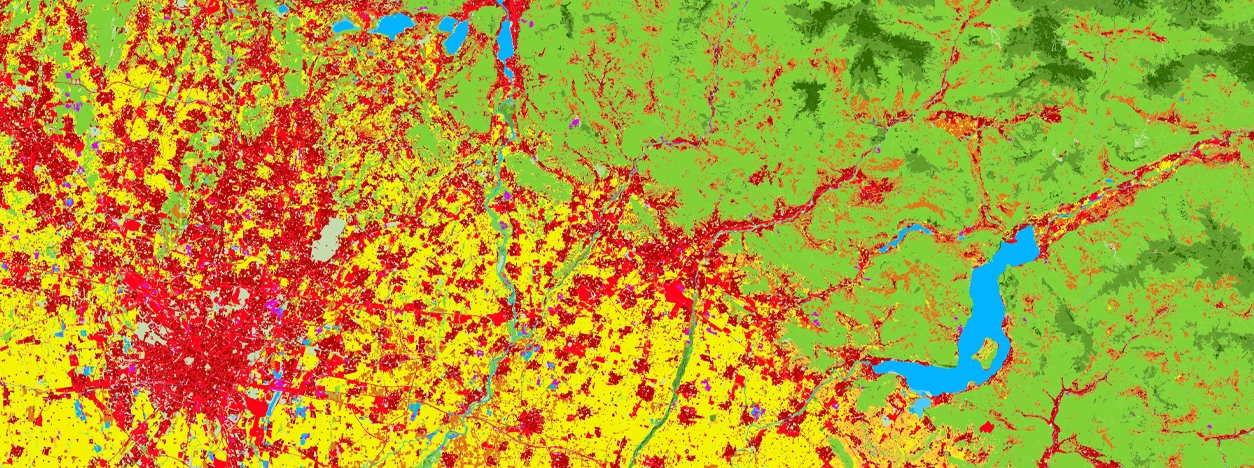
The following technical features are essential considerations when analyzing the output of land cover classification:
- Number of Categories: The land cover classification output typically consists of multiple categories representing different land cover types. These categories can vary depending on the project and the classification system used.
- For example, the CORINE Land Cover project in Europe uses a hierarchical classification system with 44 classes at the most detailed level. CORINE stands for ‘Coordination of Information on the Environment’ and is an inventory of European land cover split into different land cover classes. The project was initiated in 1985 and has been updated several times since then, with the latest update being in 2018. The CORINE Land Cover inventory is produced in cooperation with European countries and is available for download free of charge.
- In the United States, the National Land Cover Database (NLCD) uses a classification system with 16 classes. The NLCD provides nationwide data on land cover and land cover change at a 30-meter resolution based on Landsat Thematic Mapper imagery. The database is designed to provide cyclical updates on United States land cover and associated changes. The NLCD is produced by the Multi-Resolution Land Characteristics (MRLC) Consortium, a partnership of federal agencies working together to produce current, nationally consistent land cover products for all 50 states and Puerto Rico.
- Spatial Resolution: The spatial resolution refers to the size of individual pixels in the imagery data. It affects the level of detail visible in the land cover classification output. For instance, higher spatial resolution data can discern smaller features on the Earth’s surface. The appropriate operational scale depends on the spatial resolution of the satellite data used.
- Formats: The output land cover classification can be delivered in various file formats, such as GeoTIFF, ENVI, or shapefile. These formats allow for convenient integration with Geographic Information System (GIS) software for further analysis and visualization.
- Operational Scale: The operational scale of the derived land cover classification depends on the spatial resolution of the satellite data. Here’s a table providing a general correspondence between spatial resolution (in pixel size) and the most appropriate operational scale:
Spatial Resolution (Pixel Size) | Operational Scale | Map Scale | Planning Context
and Applications |
1 meter or finer | Local or site-specific | 1:2000 or finer | Detailed site analysis, urban planning, infrastructure development, precision agriculture |
3 meters | Local or site-specific | 1:6000 | Environmental impact assessment, land cover change detection in small areas |
5 meters | Local or small area | 1:10000 | Land management planning at the municipal or district level, habitat mapping, forestry management |
10 meters | Local or regional | 1:20000 | Watershed management, urban expansion monitoring, land cover change analysis at a regional scale |
30 meters | Regional or sub-regional | 1:60000 | Land use planning, regional land cover mapping, monitoring of vegetation dynamics, ecosystem assessment |
50 meters | Sub-continental or large region | 1:100000 | Land cover mapping for large-scale conservation initiatives, monitoring land degradation on a sub-continental scale |
90 meters | Sub-continental or large region | 1:180000 | Land cover mapping for large-scale conservation initiatives, monitoring land degradation on a sub-continental scale |
100 meters | Continental or global | 1:200000 or coarser | Global land cover mapping, climate change modeling, large-scale environmental monitoring |
500 meters | Continental or global | 1:1000000 or coarser | Global land cover mapping, climate change modeling, large-scale environmental monitoring |
1000 meters or coarser | Continental or global | 1:2000000 or coarser | Global land cover mapping, climate change modeling, large-scale environmental monitoring |
Note: The operational scales mentioned are indicative and can vary based on the specific context and project requirements.
RELATED LINKS AND ADDITIONAL RESOURCES
- On Map Scale and Raster Resolution – This is an ESRI blog post that discusses the relationship between map scale and raster resolution in the context of remote sensing and GIS.
- Land cover classification from Remote Sensing data – Geospatial World – This is an article from Geospatial World that provides an overview of land cover classification using remote sensing data.
- CORINE Land Cover — Copernicus Land Monitoring Service – This is the CORINE Land Cover website, which provides information on the CORINE Land Cover project in Europe. It includes sections on the project’s history, methodology, and data access.
- What is CORINE land cover? — European Environment Agency (europa.eu) – This is a page from the European Environment Agency website that provides an overview of the CORINE Land Cover project and its significance. It includes a brief description of the project and its objectives.
- National Land Cover Database | U.S. Geological Survey (usgs.gov) – This is the National Land Cover Database website, which provides information on the NLCD project in the United States. It includes sections on the project’s history, methodology, and data access.
- National Land Cover Database Class Legend and Description | Multi-Resolution Land Characteristics (MRLC) Consortium – This is a page from the MRLC Consortium website that provides a detailed description of the land cover classes used in the National Land Cover Database (NLCD). It includes a legend and descriptions of each land cover class.
- ESA WorldCover – This is the ESA WorldCover website, which provides information on the ESA WorldCover project. It includes sections on the project’s methodology, data access, and applications.
- USGS EarthExplorer – This is the USGS EarthExplorer website, which provides access to a wide range of remote sensing data, including land cover data. It includes tools for searching, previewing, and downloading data.
*Images from
https://www.nasa.gov/mission_pages/landsat/news/40th-landscapes-maricopaAZ.html