Near-surface Air Temperature
GENERAL INFORMATION
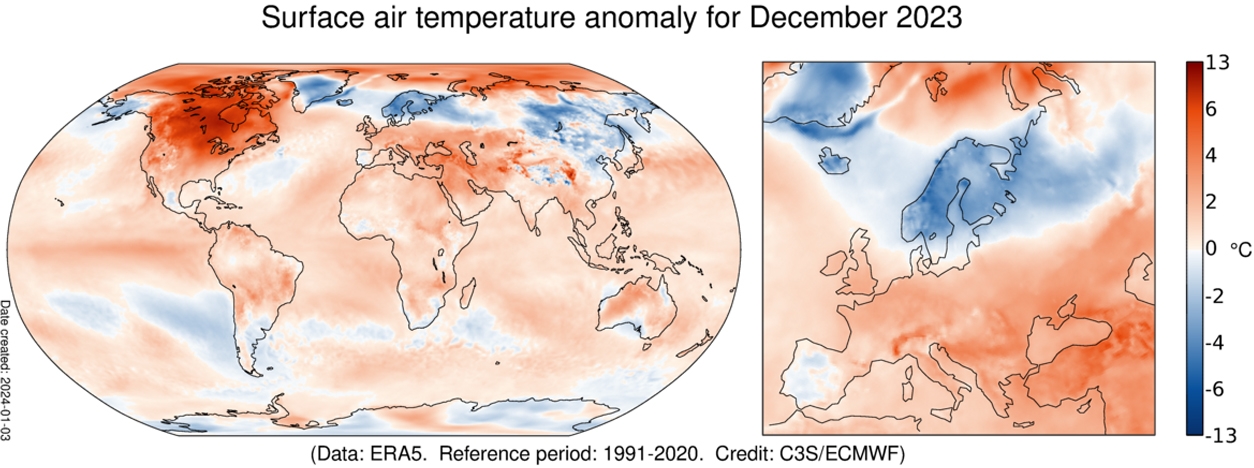
Near Surface Air Temperature (NSAT) is a vital meteorological parameter that measures the thermal state of the atmospheric layer immediately next to the Earth’s surface. It’s typically measured within a few meters from the ground, with the standard height being 2 meters above the ground or ocean surface. In simpler terms, it tells us how hot the air is near the surface of the Earth at a height of 2 meters.
NSAT, beyond being a measure of heat, is a crucial indicator of diurnal and nocturnal temperature variations and the impacts of macro-scale weather systems. It’s a fundamental variable in many scientific fields, influencing ecological systems, agricultural practices, and urban climates. It’s also a vital tool for scientists to communicate air temperatures to the public, playing a pivotal role in our comprehension of environmental processes.
NSAT plays a pivotal role in understanding and deciphering various environmental processes, encompassing climate dynamics, atmospheric phenomena, and the intricate interplay between natural and anthropogenic factors. By providing insights into temperature distributions and gradients near the Earth’s surface, NSAT serves as a valuable tool for unraveling weather patterns, assessing climatic trends, and discerning the multifaceted impacts of urbanization on local and regional climates.
NSAT is a cornerstone in climate change research, a key indicator for tracking long-term temperature trends, detecting anomalies, and understanding spatial and temporal variations in temperature regimes. By monitoring NSAT patterns and fluctuations over time, scientists can gain crucial insights into the complex dynamics of global warming, climate variability, and the far-reaching effects of human-induced greenhouse gas emissions on the Earth’s climate system.
In essence, NSAT emerges as a critical nexus point wherein the realms of meteorology, climatology, ecology, and urban planning converge, underscoring its paramount significance in elucidating the intricacies of the Earth’s climate system and informing evidence-based decision-making aimed at fostering resilience and sustainability in the face of ongoing environmental challenges.
MAIN SOURCES OF DATA
- Satellite Observations: Agencies like NASA (National Aeronautics and Space Administration) and ESA (European Space Agency) utilize satellite-based instruments such as MODIS (Moderate Resolution Imaging Spectroradiometer) and Sentinel series to monitor NSAT globally. These platforms provide extensive coverage and high temporal resolution, enabling continuous observation of temperature dynamics.
- Ground-Based Weather Stations: Meteorological stations operated by national agencies, research institutions, and organizations like NOAA (National Oceanic and Atmospheric Administration) contribute valuable ground-level NSAT data. These stations are distributed locally in territories, providing localized measurements and long-term climate records.
- Remote Sensing Technologies: Remote sensing techniques, including infrared thermography and LiDAR (Light Detection and Ranging), offer non-contact methods for measuring/modelling NSAT. These technologies are beneficial for assessing urban heat islands and monitoring temperature variations in inaccessible or challenging terrains.
- Climate Models: Numerical climate models, such as those developed by the Copernicus Climate Change Service (C3S), incorporate observational data and physical principles to simulate NSAT patterns at different spatial and temporal scales. These models play a significant role in projecting future climate scenarios and assessing the impacts of greenhouse gas emissions.
The Global Surface Temperature Analysis (GISTEMP), conducted by NASA’s Goddard Institute for Space Studies, amalgamates land surface air temperatures, predominantly from the fourth version of the Global Historical Climatology Network-Monthly (GHCN-M), with Sea Surface Temperatures (SSTs) derived from the fifth version of the Extended Reconstructed Sea Surface Temperature analysis (ERSSTv5). This integration forms an exhaustive dataset of global surface temperatures, tracing back to 1880 and updated at a monthly frequency. The data is spatially organized on a grid with a resolution of 2×2 degrees latitude-longitude. This dataset serves as a pivotal resource for tracking and studying the variability and trends in global and regional temperatures.
The European Space Agency’s Copernicus Programme provides comprehensive data on global surface temperatures through its Land Surface Temperature (LST) and Sea Surface Temperature (SST) products. The LST is the radiative skin temperature of the land surface, as measured in the direction of the remote sensor. It is estimated from top-of-atmosphere brightness temperatures in the infrared spectral channels of a constellation of geostationary satellites. The SST provides daily global sea surface temperature estimates based on observations from multiple satellite sensors. These datasets offer a spatial resolution of 5km and are updated hourly. Together, they form an integral part of the Copernicus Global Land Service, offering invaluable insights into global and regional temperature variations and trends and serving as a critical resource for climate change studies.
The Copernicus Climate Change Service (C3S) provides a dataset of near-surface meteorological variables derived from the fifth generation of the European Centre for Medium-Range Weather Forecasts (ECMWF) atmospheric reanalysis (ERA5). This dataset offers a bias-corrected reconstruction of near-surface meteorological variables from 1979 to 2019. It is intended to be used as a meteorological forcing dataset for land surface and hydrological models. The data are derived from the ERA5 reanalysis product that has been re-gridded to a half-degree resolution. Data have been adjusted using an elevation correction and monthly-scale bias corrections based on Climatic Research Unit (CRU) data (for temperature, diurnal temperature range, cloud cover, wet days number, and precipitation fields) and Global Precipitation Climatology Centre (GPCC) data (for precipitation fields only). Additional corrections are included for varying atmospheric aerosol-loading and separate precipitation gauge observations. This dataset serves as a critical resource for tracking and studying the variability and trends in global and regional temperatures and is particularly useful for climate change studies.
NSAT MODELLING
Various approaches are employed to simulate NSAT accurately, incorporating meteorological parameters, land surface characteristics, and atmospheric dynamics. It involves a diverse array of methods, ranging from physical-based simulations to empirical statistical methods and numerical weather prediction models. Each approach has its strengths and limitations, and the choice of modeling technique depends on the specific research objectives, spatial and temporal scales, and data availability.
- Physical-Based Models: These models simulate NSAT by explicitly representing the underlying physical processes governing temperature dynamics, such as thermodynamics, radiation, convection, and heat transfer. They provide detailed insights into the mechanisms driving temperature variations and require extensive input data on atmospheric conditions, land surface properties, and terrain characteristics. Physical-based models are like detailed simulations of the real world, employing fundamental physical laws and equations to depict the interactions between the atmosphere and the Earth’s surface.
- Empirical Statistical Models: These models utilize historical observational data to establish statistical relationships between NSAT and various predictor variables. They employ regression analysis, machine learning techniques, and statistical algorithms to develop predictive equations that relate NSAT to factors such as solar radiation, land surface temperature, elevation, humidity, wind speed, and land cover types. Empirical statistical models are valuable for short-term predictions and can be calibrated to specific regions or time periods based on observed data patterns.
- Numerical Weather Prediction Models (NWP): These models employ mathematical equations to predict the evolution of atmospheric conditions over time. They solve a set of partial differential equations representing the dynamics of the atmosphere, including temperature, humidity, pressure, and wind fields. NWP models assimilate observational data from weather stations, satellites, and other sources to initialize and update the model simulations. NSAT is an integral output variable of NWP models and provides valuable information for weather forecasting and climate research, particularly for short-term weather predictions.
- Regional Climate Models: Regional climate models focus on simulating climate processes at a finer spatial resolution compared to global climate models. They incorporate detailed representations of land surface processes, atmospheric dynamics, and boundary conditions to simulate NSAT variations over regional domains. Regional models downscale output from global climate models to provide more localized projections of climate change impacts, including NSAT trends, extremes, and spatial patterns. These models are instrumental in assessing the impacts of climate change at a local level and informing regional adaptation strategies.
- Coupled Atmosphere-Land Surface Models: These models integrate the interactions between the atmosphere and the Earth’s surface to simulate NSAT and other surface climate variables. They capture feedback mechanisms between land surface properties (e.g., soil moisture, vegetation cover) and atmospheric processes (e.g., evaporation, sensible heat flux). Coupled atmosphere-land surface models are valuable for studying land-atmosphere interactions, feedback mechanisms, and the impacts of land surface changes on NSAT. These models provide insights into how alterations in land surface conditions can influence local temperature regimes. For example, they might be used to study how deforestation can affect local temperatures.
In summary, Physical-based models elucidate underlying processes, empirical statistical models derive insights from observed data, numerical weather prediction models offer short-term forecasting capabilities, regional climate models focus on localized impacts, and coupled atmosphere-land surface models investigate land-atmosphere interactions. Integrating these modeling approaches enhances our understanding of NSAT dynamics and aids in addressing critical challenges related to climate variability and change.
NSAT vs. LST
Near Surface Air Temperature (NSAT) and Land Surface Temperature (LST) are related but distinct parameters in climate research:
- NSAT: Represents the temperature of the air in immediate contact with the Earth’s surface. Various factors influence it, including solar radiation, surface characteristics, and atmospheric dynamics. NSAT measurements are typically obtained using thermometers placed at standard heights above the ground or modeled based on numerical climate models.
- LST: Refers to the temperature of the Earth’s surface, including both natural surfaces (e.g., soil, vegetation) and artificial surfaces (e.g., buildings, roads). LST measurements are mainly derived from satellite observations, which capture the thermal radiation emitted by the Earth’s surface. LST serves as a proxy for surface energy fluxes and land-atmosphere interactions.
While NSAT and LST are correlated, discrepancies may arise due to factors such as land cover, urbanization, and surface materials. Urban areas, for example, often exhibit much higher LST values during daytime compared to nearby rural areas due to the urban heat island effect, wherein artificial surfaces absorb and re-emit solar radiation, leading to elevated temperatures. Instead, at nighttime, NSAT and LST values tend to be closer to each other.
MAIN APPLICATIONS
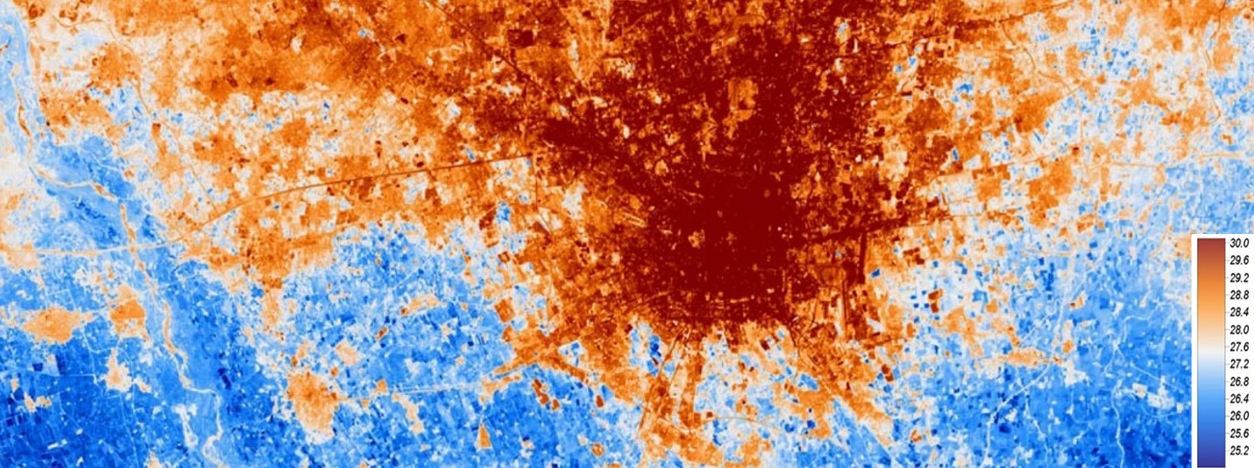
Near Surface Air Temperature plays a pivotal role in climate science, providing valuable insights into the Earth’s climate system, weather dynamics, and the impacts of anthropogenic activities. Through a combination of satellite observations, ground-based measurements, and advanced modeling techniques, researchers can analyze NSAT patterns comprehensively and address pressing challenges related to climate change and global warming. Some of the main applications include:
- Climate Change Monitoring: NSAT data are crucial for detecting long-term temperature trends and hotspots. They also play a significant role in assessing the impacts of global warming on various sectors, including ecosystems, agriculture, and human health.
- Weather Forecasting: Accurate NSAT measurements are integral to improving weather forecasting models. They enhance predictions of temperature extremes, heatwaves, and other meteorological events, contributing to more reliable and timely weather forecasts.
- Urban Planning and Management: NSAT variations within urban areas provide valuable urban planning and management insights. Understanding these variations is critical for mitigating urban heat island effects, designing climate-resilient cities, and making informed decisions about sustainable urban development.
- Ecosystem Management: NSAT data are used to assess ecosystems’ vulnerability to temperature changes. They guide conservation efforts and inform adaptive management strategies in response to climate variability, helping to maintain biodiversity and ecosystem health.
RELATED LINKS AND ADDITIONAL RESOURCES
- NASA GISS – GISTEMP: Offers access to the GISS Surface Temperature Analysis (GISTEMP), providing datasets and analysis tools for monitoring global temperature trends and understanding climate dynamics.
- UCAR Climate Data Guide – GISTEMP: Offers detailed information on GISTEMP (Global Surface Temperature Data) provided by NASA’s Goddard Institute for Space Studies (GISS).
- NASA GISS – Global Climate Modeling: Explores NASA’s projects on global climate modeling, aimed at simulating Earth’s climate system to understand past variations and predict future climate changes.
- NASA GISS – Climate Impacts: Investigates the impacts of global warming and climate change on various Earth systems, including ecosystems, water resources, and human societies. The project aims to assess risks and develop strategies for adaptation and resilience.
- NASA – Warmest Year on Record (2023): Confirms 2023 as the warmest year on record through NASA’s analysis, emphasizing the urgency of addressing climate change and its impacts.
- NASA – Climate Events of 2020: Examines the climate events of 2020 and their expression of excess heat on Earth’s surface, providing insights into extreme weather phenomena and the impacts of climate change.
- NOAA – Tools for Weather Forecasting: Explores six tools utilized by meteorologists at the National Oceanic and Atmospheric Administration (NOAA) for weather forecasting, essential for predicting short-term weather patterns and enhancing disaster preparedness and response efforts.
- NOAA NCEI – Global Historical Climatology Network Monthly: Provides access to the Global Historical Climatology Network Monthly dataset, curated by the National Centers for Environmental Information (NCEI) of NOAA.
- NOAA NCEI – Metadata for Dataset: Provides metadata information for a specific dataset available from the National Centers for Environmental Information (NCEI) of NOA.
- NOAA Ocean Service – Sea Surface Temperature Facts: Presents facts and information about sea surface temperature (SST) provided by the National Oceanic and Atmospheric Administration (NOAA) Ocean Service.
- NOAA NCEI – Extended Reconstructed Sea Surface Temperature (SST): Provides access to the Extended Reconstructed Sea Surface Temperature (SST) dataset, curated by the National Centers for Environmental Information (NCEI) of NOAA.
- Copernicus Land Monitoring Service – Land Surface Temperature (LST): Offers access to the Land Surface Temperature (LST) dataset provided by the Copernicus Land Monitoring Service.
- Copernicus Services Catalogue – Derived Near-Surface Meteorological Variables: Provides access to derived near-surface meteorological variables through the Copernicus Services Catalogue.
- Copernicus Climate Data Store – Derived Near-Surface Meteorological Variables: Offers access to a portfolio of datasets containing derived near-surface meteorological variables through the Copernicus Climate Data Store.
- Copernicus Climate Data Store – Derived Near-Surface Meteorological Variables Overview: Provides an overview of the dataset containing derived near-surface meteorological variables available through the Copernicus Climate Data Store (CDS). Includes essential variables such as temperature, humidity, wind speed, and precipitation.
- Copernicus Climate Data Store – Derived Near-Surface Meteorological Variables Form: Copernicus Climate Data Store (CDS) for the dataset containing derived near-surface meteorological variables. Facilitates customized access to relevant meteorological data for research and analysis purposes.
- Copernicus Earth System Science Data – Article: Provides access to an article published in Copernicus Earth System Science Data, offering detailed information and datasets related to Earth system science.
- Estimation of Surface and Near-Surface Air Temperatures: Estimates NSAT using multiple linear regression models (MLR) and random forest (RF) algorithms.
- All-Weather Near-Surface Air Temperature Estimation Based on Satellite Data: Scientific paper that presents a novel model for estimating daily 1-km all-weather near-surface air temperature based on machine learning techniques.
- Estimating day and night instantaneous near-surface air temperature: Scientific paper based on empirical statistical modelling to estimate NSAT.
*Images from
https://climate.copernicus.eu/surface-air-temperature-december-2023